The Evolution of AI in Academic Research
From Manual to Automated: A Brief History
The traditional research process has long been time-consuming and labor-intensive. Researchers spent countless hours sifting through physical libraries, manually cross-referencing sources, and painstakingly organizing findings. This approach, while thorough, was often limited by human capacity for information processing and physical constraints of accessing resources.
The advent of digital technologies brought the first wave of change, with online databases and search engines making it easier to access information. However, core tasks of analysis, synthesis, and knowledge organization still relied heavily on manual effort. Early attempts at digitization and automation focused primarily on improving access to information rather than revolutionizing how that information was processed and understood.
The AI Revolution in Research Methodologies
The introduction of machine learning and natural language processing has marked a paradigm shift in research methodologies. These AI technologies have transformed how we approach data analysis and literature reviews, bringing unprecedented speed and efficiency to the research process.
Machine learning algorithms can now process vast amounts of data, identifying patterns and connections that might elude human researchers. Natural language processing enables AI systems to understand and interpret academic texts, extracting key information and even generating summaries. This AI-driven approach is not just faster; it's opening up new possibilities for cross-disciplinary insights and comprehensive literature analysis that were previously impractical due to time and cognitive limitations.
Key Features of Leading AI Research Assistants
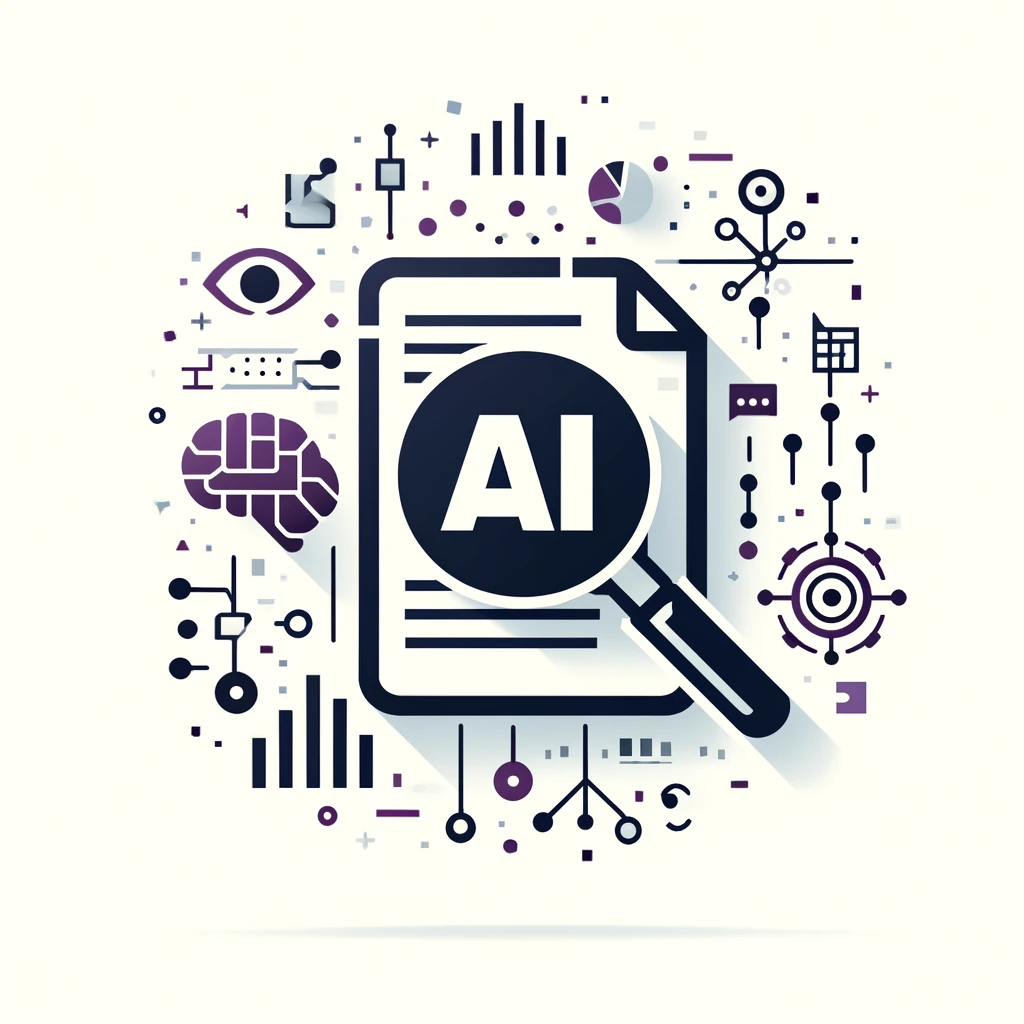
Intelligent Document Analysis and Summarization
One of the most powerful features of modern AI research assistants is their ability to perform intelligent document analysis and summarization. These tools can rapidly process academic papers, reports, and other research materials, automatically extracting key concepts, methodologies, and findings. This capability dramatically reduces the time researchers spend on initial reading and note-taking.
The summarization function goes beyond simple text extraction. Advanced AI algorithms can generate concise, accurate summaries that capture the essence of complex documents. These summaries are often structured to highlight the most important elements, such as research questions, methodologies, results, and conclusions. This allows researchers to quickly grasp the core ideas of numerous papers, enabling them to cover more ground in their literature reviews and identify relevant sources more efficiently.
Advanced Natural Language Query Systems
Another game-changing feature of top AI research assistants is their advanced natural language query systems. These systems can understand and respond to complex research questions, going far beyond simple keyword matching. They interpret the context and intent behind queries, providing more relevant and nuanced responses.
The contextual understanding of these AI systems allows them to engage in a more natural, conversational interaction with researchers. You can ask follow-up questions, request clarifications, or explore related topics, and the AI will maintain context throughout the conversation. This capability is particularly valuable when exploring interdisciplinary topics or when you're venturing into unfamiliar research territories.
Top AI Research Assistants Revolutionizing Academia
Comprehensive Comparison of Popular Platforms
To help you navigate the landscape of AI research assistants, let's compare some of the most popular platforms:
Platform | Key Features | Best For | User Satisfaction |
---|---|---|---|
ResearchFlow | Interactive knowledge maps, multi-document comparison | Researchers, students, analysts | 4.8/5 |
Elicit | Literature review automation, study design assistance | Academic researchers | 4.5/5 |
Semantic Scholar | AI-powered academic search engine, citation analysis | Literature reviews, trend analysis | 4.3/5 |
Specialized AI Tools for Different Research Domains
The AI research assistant landscape also includes tools tailored to specific research domains:
STEM fields: Platforms like Sci-AI offer specialized features for handling scientific data, mathematical equations, and complex experimental designs
Social sciences: Tools like MAXQDA incorporate AI to assist with qualitative data analysis, coding, and mixed-methods research
Humanities: AI assistants like Voyant Tools provide text analysis capabilities tailored to literary and historical research
These domain-specific tools often integrate seamlessly with existing research workflows, enhancing productivity without disrupting established methodologies.
Maximizing Efficiency with AI Research Assistants
Strategies for Effective AI-Human Collaboration
To get the most out of AI research assistants, it's crucial to develop strategies for effective collaboration:
Formulate clear, specific queries
Use iterative questioning to refine results
Combine AI insights with critical thinking
Validate AI-generated information against reliable sources
Maintain a healthy skepticism and always cross-reference findings
Remember, AI assistants are powerful tools, but they're not replacements for human critical thinking. Use them to augment your research process, not to drive it entirely.
Overcoming Common Challenges and Limitations
While AI research assistants offer tremendous benefits, they also come with challenges that need to be addressed. Researchers should be aware of potential biases in AI-generated content, ensure accuracy by cross-referencing information, and prioritize data privacy and security when using these tools.
The Future of AI in Research: Trends and Predictions
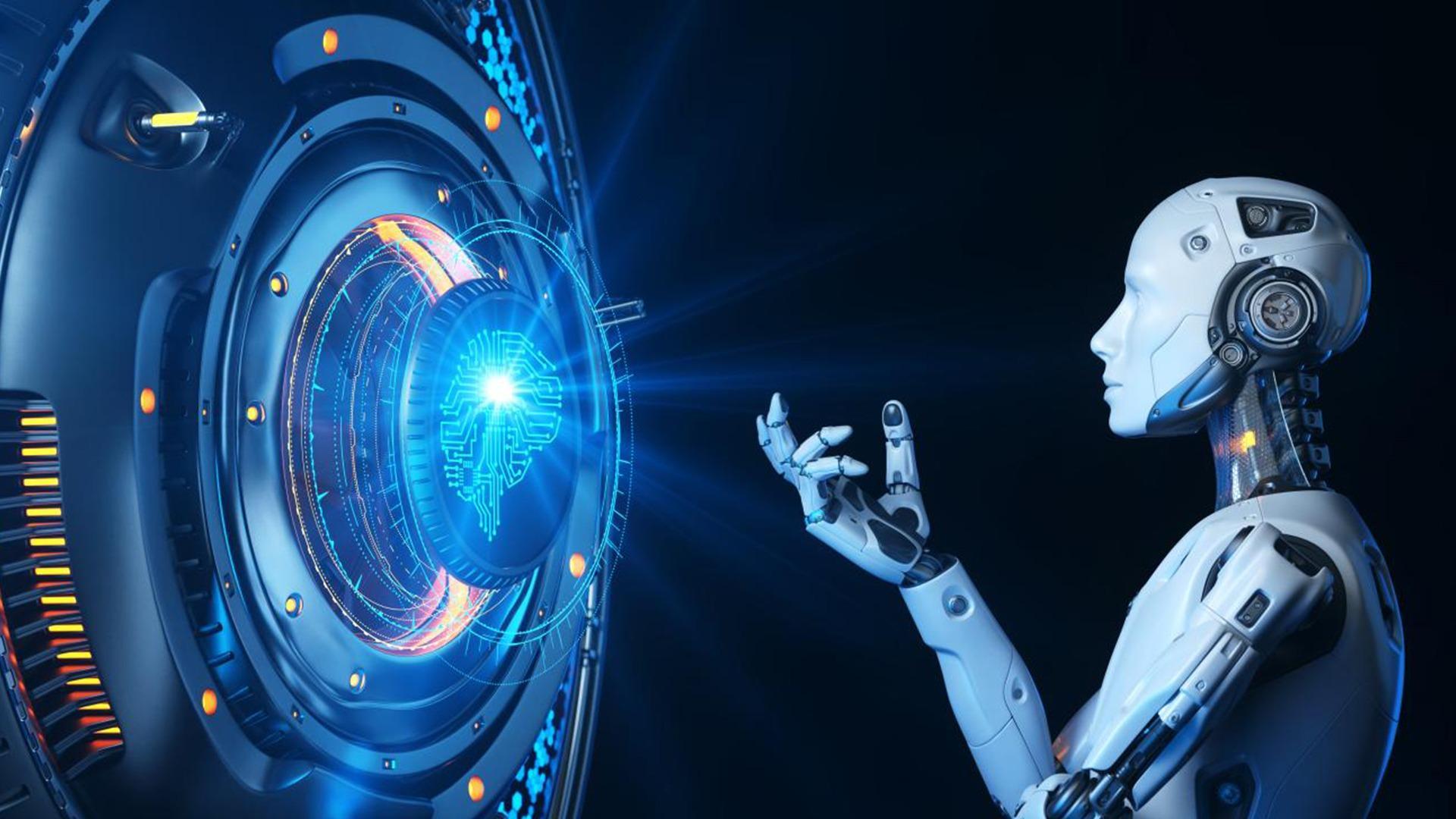
Emerging Technologies Shaping the Research Landscape
The future of AI in research looks incredibly promising, with several emerging technologies poised to reshape the landscape. Advancements in natural language processing will enable more nuanced understanding of complex academic texts. Machine learning models will adapt to individual researchers' needs and preferences. The potential impact of quantum computing may enhance AI processing power for larger datasets and more complex analyses.
How ResearchFlow is Leading the AI Research Revolution
ResearchFlow is at the forefront of this AI research revolution, pioneering a unique approach to knowledge mapping and visualization. By converting complex research papers into interactive knowledge maps, ResearchFlow transforms how researchers interact with information. This visual approach allows for a more intuitive understanding of complex topics, enabling researchers to grasp key ideas and connections much faster than traditional methods.
The integration of cutting-edge AI technology in ResearchFlow provides comprehensive research support that goes beyond simple information retrieval. Its AI can analyze multiple documents simultaneously, identifying patterns, contradictions, and potential research gaps across a broad body of literature. This multi-document comparison feature is particularly powerful for interdisciplinary research, where insights often emerge from the intersection of different fields.
By leveraging these advanced AI research assistants, particularly platforms like ResearchFlow, researchers can significantly streamline their processes, saving countless hours and uncovering insights that might otherwise remain hidden. As we look to the future, the symbiosis between human intellect and AI assistance promises to accelerate the pace of discovery and innovation across all fields of study.