The Challenge of Research Synthesis
Time-Consuming Traditional Methods
Synthesizing research findings has long been a daunting task for academics and professionals alike. Traditional methods of research synthesis often involve manual note-taking and organization, which can be incredibly time-consuming and prone to human error. As researchers, we've all experienced the frustration of flipping through stacks of papers, trying to connect the dots between various studies and their findings. This process not only eats up valuable time but also makes it challenging to maintain a comprehensive overview of the subject matter.
The difficulty in connecting ideas across multiple papers is particularly pronounced when dealing with interdisciplinary research or when trying to identify emerging trends in a rapidly evolving field. These manual methods can lead to missed connections and insights, potentially impacting the quality and depth of our research outcomes.
Information Overload in Academic Research
The exponential growth of published papers in recent years has created a veritable tsunami of information for researchers to navigate. It's not uncommon to find thousands of papers on a single topic, making it increasingly difficult to stay current with the latest findings in any given field. This information overload presents a significant challenge for researchers trying to synthesize findings effectively.
The struggle to stay abreast of cutting-edge developments while also conducting our own research can lead to burnout and diminished productivity. Moreover, the sheer volume of information can make it challenging to identify truly groundbreaking studies amidst the noise, potentially leading to missed opportunities for innovation and advancement in our respective fields.

Strategies for Efficient Research Synthesis
Systematic Literature Review Techniques
Systematic literature review techniques have emerged as a powerful tool for managing the complexities of research synthesis. The PRISMA (Preferred Reporting Items for Systematic Reviews and Meta-Analyses) method, in particular, has gained widespread adoption across various disciplines. This approach provides a structured framework for identifying, screening, and analyzing relevant studies, ensuring a comprehensive and unbiased review of the literature.
The PRISMA method typically involves several key steps:
Defining clear research questions and criteria
Conducting a thorough database search
Screening studies based on predefined inclusion/exclusion criteria
Extracting and analyzing data from selected studies
Synthesizing findings and assessing the quality of evidence
To manage large volumes of papers effectively, researchers can leverage tools specifically designed for systematic reviews, including reference management software, screening and data extraction tools, and meta-analysis software.
Leveraging Technology for Faster Synthesis
The advent of AI-powered text analysis tools has revolutionized the way we approach research synthesis. These sophisticated algorithms can rapidly process vast amounts of text, identifying key themes, methodologies, and findings across multiple papers. Some of the ways AI can accelerate synthesis include:
Automated summarization of research papers
Identification of common themes and research gaps
Extraction of key statistics and data points
Generation of literature review drafts
Visualization software for concept mapping is another technological advancement that's transforming research synthesis. These tools allow researchers to create interactive visual representations of complex ideas and relationships between different studies, enhancing understanding and leading to more insightful analyses and conclusions.
Accelerating Synthesis with AI-Assisted Tools
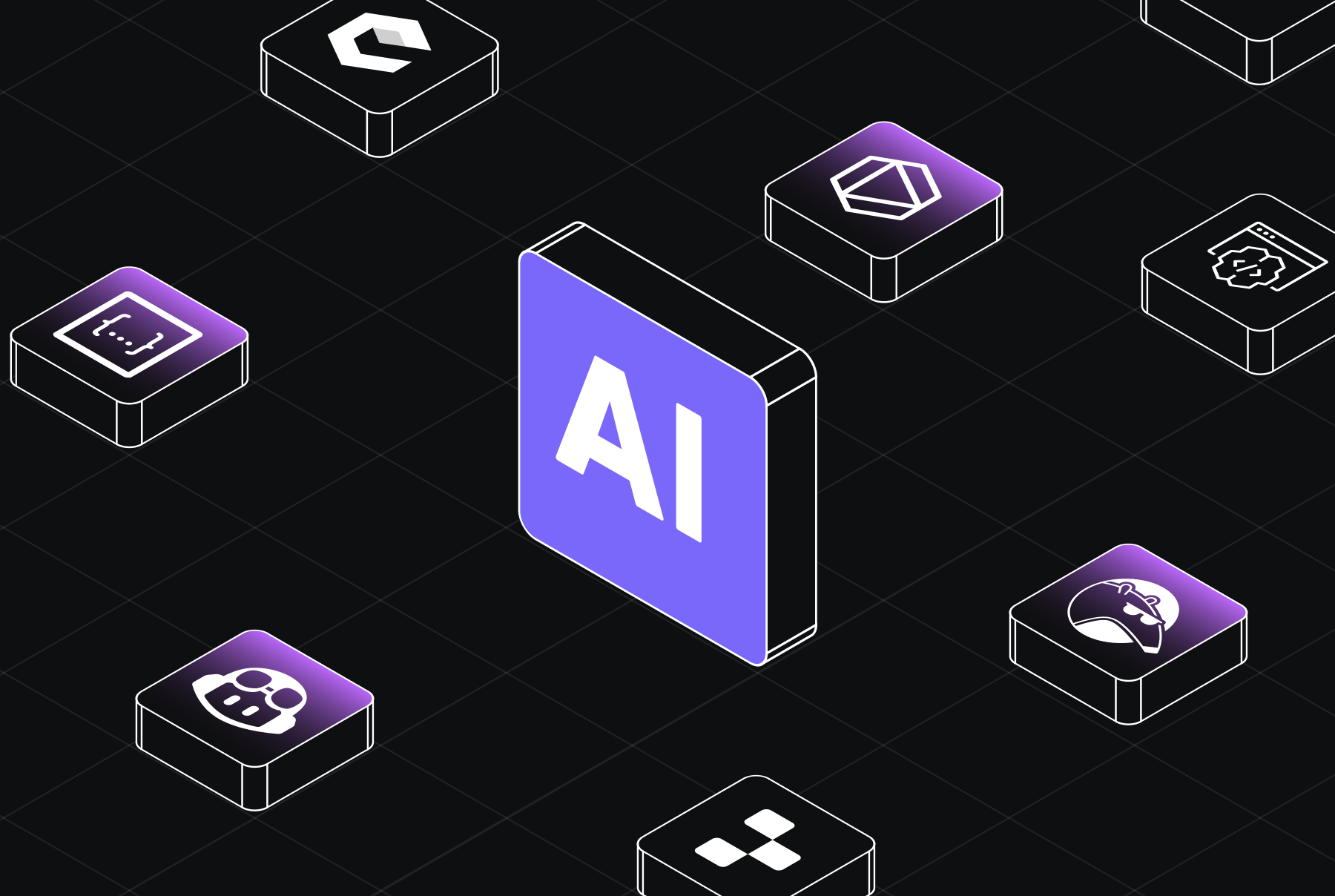
Machine Learning for Pattern Recognition
Machine learning algorithms have become indispensable in the quest for faster and more accurate research synthesis. These powerful tools excel at automated identification of key themes across large datasets of academic literature. By training on vast corpora of scientific papers, ML models can quickly discern recurring concepts, methodologies, and findings that might take a human researcher weeks or even months to uncover manually.
Cross-referencing similar concepts across papers is another area where machine learning shines. AI algorithms can identify subtle connections between studies that might not be immediately apparent to the human eye. This capability is particularly valuable when dealing with interdisciplinary research or when trying to bridge gaps between seemingly unrelated fields.
Natural Language Processing in Research
Natural Language Processing (NLP) has emerged as a powerful ally in the realm of research synthesis. By leveraging advanced NLP techniques, researchers can now extract main ideas and methodologies from academic papers with unprecedented speed and accuracy. These AI-powered tools can analyze the structure and content of research papers, identifying key sections such as hypotheses, methodologies, results, and conclusions.
Summarizing complex academic language is another area where NLP excels. NLP algorithms can distill dense texts into more accessible summaries, highlighting the most salient points and findings. This capability is particularly useful when dealing with large volumes of literature or when researchers need to quickly grasp the essence of papers outside their immediate area of expertise.
ResearchFlow: Revolutionizing Research Synthesis
AI-Powered Knowledge Mapping
ResearchFlow's AI-powered knowledge mapping feature represents a significant leap forward in research synthesis technology. The platform's ability to convert PDFs into interactive knowledge maps with just one click transforms dense academic papers into visually intuitive structures. The interactive nature of these knowledge maps allows for dynamic exploration of ideas, enabling researchers to dive deeper into specific areas of interest or zoom out for a broader perspective.
The multi-document comparison feature of ResearchFlow takes synthesis to the next level by enabling quick insight generation across multiple papers. This tool allows researchers to visually compare and contrast findings, methodologies, and conclusions from different studies side by side, significantly reducing the time and cognitive load required to synthesize information from diverse sources.
Integrated Workflow for Seamless Synthesis
ResearchFlow's integrated workflow combines search, reading, and note-taking into a single, cohesive platform, streamlining the entire research process. This unified approach eliminates the need to juggle multiple tools and applications, reducing cognitive overhead and allowing researchers to maintain focus on their analysis.
The AI-assisted mind mapping feature for flexible knowledge organization is another standout aspect of ResearchFlow's integrated workflow. This tool allows researchers to visually organize their thoughts, ideas, and findings in a dynamic and adaptable manner. The AI component suggests relevant connections and helps identify potential gaps in the researcher's knowledge structure, promoting a more comprehensive and nuanced understanding of complex research topics.
Implementing Advanced Synthesis Techniques
Best Practices for Using AI in Research
When incorporating AI into the research synthesis process, it's crucial to strike a balance between leveraging AI assistance and maintaining critical thinking. Here are some best practices for using AI in research:
Use AI for initial data gathering and pattern recognition
Critically evaluate AI-generated insights and cross-reference with primary sources
Combine AI-assisted analysis with traditional research methods for a comprehensive approach
Regularly update and refine AI models with the latest research data
Maintain transparency about the use of AI in your research methodology
Ensuring accuracy and avoiding bias in AI-generated summaries is paramount to maintaining the integrity of your research. Researchers should regularly cross-check AI-generated summaries against original sources, use multiple AI tools for consistency, and involve human experts in reviewing and validating AI-generated insights.
Measuring the Impact on Research Productivity
To truly understand the value of advanced synthesis tools like ResearchFlow, it's essential to quantify their impact on research productivity. Several case studies have demonstrated significant improvements in both efficiency and depth of understanding. Here's a summary of key metrics from various case studies:
Metric | Traditional Methods | Improvement | |
---|---|---|---|
Time to complete literature review | 120 hours | 72 hours | 40% reduction |
Number of papers processed per day | 5-7 | 15-20 | 200% increase |
Depth of cross-disciplinary insights | Moderate | High | Significant improvement |
Time to identify research gaps | 4-6 weeks | 1-2 weeks | 66% reduction |