The Evolution of Scholarly Research in the Digital Age
From Manual to Automated: A Brief History
The landscape of scholarly research has undergone a dramatic transformation in recent decades. The transition from traditional research methods to digital tools has been revolutionary, opening up new possibilities for researchers to explore vast amounts of data quickly and efficiently.
Key milestones in the development of research technologies include:
Launch of academic search engines like Google Scholar in 2004
Rise of open-access journals and repositories like arXiv
Integration of artificial intelligence into the research process
These developments have laid the groundwork for the next big leap in scholarly research.
The Rise of AI in Academic Research
The introduction of machine learning and natural language processing into academic research has been a game-changer. AI technologies are now capable of analyzing vast amounts of text, identifying patterns, and extracting insights at an unprecedented scale.
AI is reshaping how we conduct research by:
Processing and categorizing research papers
Automating summarization of complex academic texts
Suggesting research directions
Identifying gaps in current knowledge
Assisting in the writing process
Unleashing the Power of AI in Scholarly Analysis
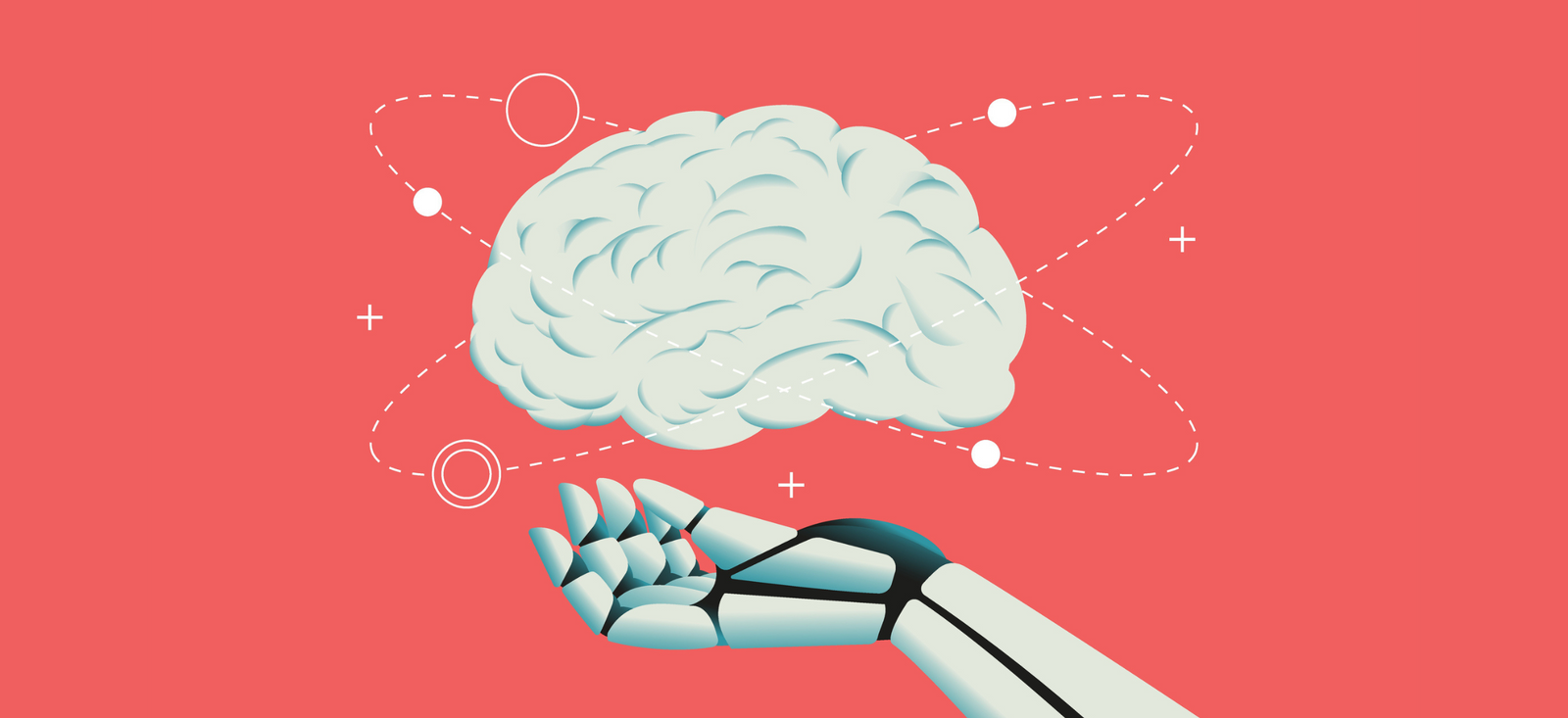
AI-Driven Literature Review and Synthesis
AI has revolutionized the literature review process, turning a weeks-long task into one that can be accomplished in hours or even minutes. AI algorithms can now analyze thousands of papers across multiple databases, identifying key themes, methodologies, and findings.
Benefits of AI in literature review include more comprehensive reviews, identification of patterns and connections across studies, uncovering hidden relationships between seemingly unrelated research, and generating new insights and research directions.
Enhanced Data Extraction and Visualization
AI tools have emerged as powerful allies in data extraction and visualization. These tools can parse through dense research papers, extracting key data points with remarkable accuracy. Once extracted, AI can assist in organizing this data into structured formats and create interactive visualizations.
ResearchFlow: Revolutionizing Scholarly Research with AI
Transforming Papers into Interactive Knowledge Maps
ResearchFlow's innovative approach turns papers into structured knowledge maps with just one click. This feature analyzes content, identifies key concepts, and organizes them into an interactive map, providing a bird's-eye view of the entire paper.
AI-Powered Multi-Document Comparison
ResearchFlow's AI-powered multi-document comparison tool addresses one of the most time-consuming aspects of academic research: synthesizing information from multiple sources. This feature allows researchers to upload several papers on a similar topic and let the AI identify similarities and differences across them.
Maximizing Research Efficiency with AI-Assisted Workflows
Streamlining the Research Process
ResearchFlow exemplifies streamlined research with its integrated approach, combining search, reading, and note-taking functionalities into a single, cohesive workflow. The platform offers AI-powered search functionality, in-platform reading with AI-powered highlighting and summarization, AI-guided questioning for deeper exploration, and an AI-assisted note-taking system.
Collaborative Features for Team-Based Research
ResearchFlow offers powerful features for team-based research that leverage AI to enhance collaboration, including real-time sharing and editing of knowledge maps, AI-facilitated cross-disciplinary connections, version control and change tracking, and a clear trail of research development.
The Future of Scholarly Research AI: Trends and Predictions
Emerging Technologies in Academic AI
Several exciting trends and emerging technologies promise to further revolutionize scholarly research AI:
Advancements in natural language understanding and generation
Development of more sophisticated semantic understanding in AI
Integration of AI with quantum computing
AI's ability to work with multimodal data
Ethical Considerations and Best Practices
As AI becomes increasingly integrated into scholarly research, it's crucial to address ethical considerations and establish best practices for its use.
Ethical Consideration | Best Practice | Importance |
---|---|---|
Bias in AI systems | Regularly audit AI tools for bias and use diverse training data | Ensures fairness and accuracy in research outcomes |
Transparency in AI use | Clearly document AI tools and methods used in research | Maintains research integrity and reproducibility |
Data privacy and security | Use secure, compliant AI platforms and anonymize sensitive data | Protects research subjects and confidential information |
Over-reliance on AI | Combine AI insights with human expertise and critical thinking | Balances efficiency with depth of analysis |
The future of scholarly research AI is bright, but it requires active engagement to ensure it enhances, rather than compromises, the quality and integrity of academic research. As researchers, it's our responsibility to harness the power of AI while remaining true to the fundamental principles of scholarly inquiry.