How to Build Data Infrastructure for Research [5 Simple Steps]

Understanding the Foundations of Research Data Infrastructure
Key Components of Effective Data Management
In the rapidly evolving world of research, data infrastructure forms the backbone of efficient and impactful studies. Data infrastructure in a research context is the comprehensive framework that enables researchers to collect, store, process, and analyze vast amounts of information seamlessly.
The essential elements of a robust research data infrastructure include:
Storage solutions
Processing capabilities
Security measures
Accessibility features
These components work in tandem to create a cohesive ecosystem where data can flow freely yet securely. Imagine a well-oiled machine where each part plays a crucial role in transforming raw data into actionable insights. That's the power of an effective data infrastructure.
Challenges in Traditional Research Data Handling
While the importance of data in research is undeniable, traditional methods of data handling often fall short in today's fast-paced academic environment. Manual data organization, once the norm, now presents significant limitations. Researchers frequently find themselves drowning in a sea of spreadsheets, struggling to maintain consistency and accuracy across their datasets.
Moreover, the issue of data silos and fragmented information plagues many research institutions. Valuable data is often scattered across different departments, locked away in incompatible formats or inaccessible systems. This fragmentation not only hampers collaboration but also leads to duplication of efforts and missed opportunities for groundbreaking discoveries.
Designing a Robust Data Architecture for Research Projects
Selecting the Right Data Storage Solutions
When it comes to building a solid foundation for your research data infrastructure, choosing the right storage solution is paramount. The debate between cloud-based and on-premise storage continues in academic circles, with each option offering distinct advantages.
cloud-based storage solutions provide unparalleled flexibility and accessibility. Researchers can access data from anywhere in the world, collaborate with international colleagues in real-time, and scale storage needs effortlessly as projects grow. On-premise storage offers greater control over data and can be preferable for highly sensitive research subjects.
Considerations for scalability and data growth should be at the forefront of your decision-making process. Research projects have a tendency to expand rapidly, often in unexpected directions. Your chosen storage solution should accommodate this growth without causing disruptions or requiring frequent overhauls.
Implementing Data Integration and Interoperability
In the complex world of research, data rarely exists in isolation. The true power of your data infrastructure lies in its ability to connect and communicate across different sources and systems. Implementing effective strategies for connecting disparate data sources is crucial for painting a complete picture of your research landscape.
One key aspect of this integration is the importance of standardized data formats and APIs (Application Programming Interfaces). These act as a common language, allowing different systems to communicate seamlessly. By establishing these standards, you're essentially creating a universal translator for your research data.
Leveraging AI and Machine Learning in Research Data Infrastructure
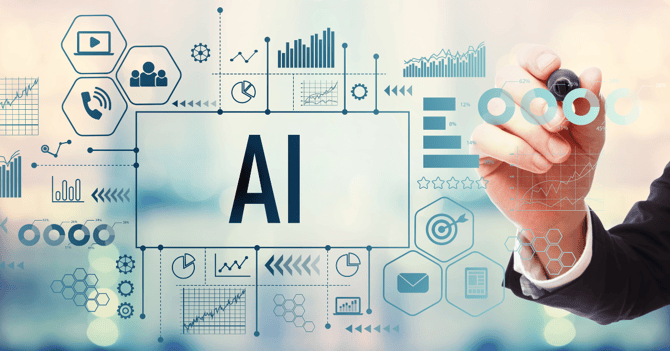
Enhancing Data Analysis with AI-Powered Tools
The integration of AI into research data infrastructure is revolutionizing the way we extract insights from complex datasets. Tools like ResearchFlow are at the forefront of this transformation, offering AI capabilities that can significantly accelerate the research process. These advanced systems can sift through mountains of data, identifying patterns and connections that might elude even the most diligent human researcher.
One of the most exciting benefits of AI-powered analysis is automated data extraction and summarization. This not only saves countless hours of manual review but also ensures that crucial information isn't overlooked. It's like having a tireless research assistant who can work 24/7, digesting information at superhuman speeds.
Machine Learning for Predictive Research Insights
The application of machine learning in research goes beyond simple data analysis. It opens up new frontiers in predictive insights, potentially reshaping how we approach scientific inquiry. In the realm of research trend forecasting, ML algorithms can analyze vast amounts of published literature, grant proposals, and conference proceedings to identify emerging areas of interest or potential breakthroughs.
AI assists in hypothesis generation and testing by analyzing patterns across diverse datasets. Machine learning models can suggest novel hypotheses that human researchers might not have considered. This doesn't replace human creativity but rather augments it, providing a springboard for innovative thinking.
Ensuring Data Security and Compliance in Research Infrastructure
Implementing Robust Data Protection Measures
In the world of research, data is gold β and like any valuable asset, it needs robust protection. Implementing strong data protection measures is essential for maintaining the integrity and credibility of your research. Best practices for data encryption and access control form the first line of defense against potential breaches or unauthorized access.
Strategies for securing sensitive research information go beyond just technical measures. It involves creating a culture of security awareness among your research team. This might include:
Regular training sessions
Clear protocols for handling sensitive data
Simulated security breaches to test system resilience
Ongoing security audits and updates
Remember, your data infrastructure is only as secure as its weakest link β often the human element.
Navigating Regulatory Requirements for Research Data
The landscape of data protection regulations is complex and ever-changing, especially in the research world where data often crosses international borders. Key regulations like GDPR in Europe and HIPAA in the United States set stringent standards for how personal and sensitive data should be handled.
Ensuring compliance with these regulations while maintaining research efficiency requires a deep understanding of the legal requirements and how they apply to your specific research context. This might involve conducting regular audits, maintaining detailed documentation of your data handling processes, and potentially working with legal experts to navigate the more complex aspects of data law.
Optimizing Research Workflows with Advanced Data Infrastructure
Streamlining Data Collection and Processing
Efficient data collection and processing are the cornerstones of productive research. Advanced tools like ResearchFlow are revolutionizing this aspect of research workflow. With features like one-click PDF upload and automated knowledge mapping, these tools can transform a time-consuming manual process into a streamlined, efficient operation.
Automating data cleaning and preprocessing tasks can eliminate hours of tedious work, allowing researchers to focus on analysis and interpretation rather than data wrangling.
Enhancing Collaboration and Knowledge Sharing
In today's interconnected research landscape, collaboration is key to groundbreaking discoveries. Advanced data infrastructure plays a crucial role in facilitating this collaboration. Features for multi-document comparison and insights allow researchers to easily identify similarities and differences across various sources, fostering a more comprehensive understanding of their field.
Creating a centralized knowledge hub for research teams is another game-changing aspect of modern data infrastructure. This acts as a single source of truth, where team members can access shared resources, track project progress, and contribute their findings in real-time.
Feature | Description | Benefit |
---|---|---|
AI-Powered Analysis | Automated extraction and summarization of key information from research papers | Saves time, ensures comprehensive coverage of literature |
Multi-Document Comparison | Tools for comparing and contrasting multiple research documents | Facilitates deeper understanding and identification of research gaps |
Centralized Knowledge Hub | A unified platform for storing and accessing all research-related information | Enhances collaboration and ensures all team members are on the same page |